Document type : article published in Computers and Electronics in Agriculture
Authors: Alvaro Fuentes, Sook Yoon, Jongbin Park, Dong SunPark
Preview: Behavior is an important indicator for understanding the well-being of animals. This process has been frequently carried out by observing video records to detect changes with statistical analysis, or by using portable devices to monitor animal movements. However, regarding animal welfare, the use of such devices could affect the normal behavior of the animal, and present limitations in its applicability. This paper introduces an approach for hierarchical cattle behavior recognition with spatio-temporal information based on deep learning. Our research extends the idea of activity recognition in video and focuses specifically on cattle behavior. Our framework involves appearance features at frame-level and spatio-temporal information that incorporates more context-temporal features. The system can detect (class) and localize (bounding box) regions containing multiple cattle behaviors in the video frames. Additionally, we introduce our cattle behavior dataset that includes videos recorded with RGB cameras on different livestock farms during day and night environments. Experimental results show that our system can effectively recognize 15 different types of hierarchical activities divided into individual and group activities, and also part actions. Qualitative and quantitative evaluation evidence the performance of our framework as an effective method to monitor cattle behavior.
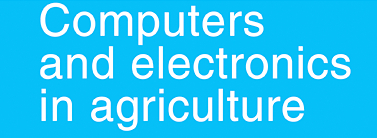