Document type : scientific article published in Computers and Electronics in Agriculture
Authors: Xuqiang Yin, Dihua Wu, Yuying Shang, Bo Jiang, Huaibo Song
Preview: Accurate and rapid recognition of dairy cow's motion behaviours is the key to intelligent perception of its health state. To achieve the recognition of cows' lying, standing, walking, drinking and feeding behaviours, first, based on the advantage of the efficient feature extraction of EfficientNet, the spatial feature extraction of cow's video frames was realized. Then, to fully extract the characteristics of different behaviour information of dairy cows, the BiFPN (bidirectional feature pyramid network) was used to realize the efficient fusion of characteristics in the 3-5 layers of EfficientNet. Finally, the behaviour information was sent to the BiLSTM (bidirectional long short-term memory) module, which integrates the attention mechanism to realize the aggregation of video frames in a time series, thus realizing fast and accurate recognition of dairy cow's motion behaviours. 1009 videos containing 2,270,250 frames of dairy cows in different scenes and postures were collected and tested to compare the performance of the proposed algorithm, four state-of-the-art behaviour recognition algorithms: C3D, VGG16-LSTM, ResNet50-LSTM, and DensNet169-LSTM were carried out. Meanwhile, the precision, recall, accuracy, recall and the average number of frames recognized per second (ANFR) were used to evaluate the performance of the algorithm. Experimental results showed that the behaviour recognition accuracy of the algorithm was 97.87%, which was 4.25% higher than that of the classical ResNet50-LSTM, and the ANFR was 134f/s. In addition, the study was combined with a sliding window to realize the behaviour recognition of undivided single-target dairy cow videos, and the final recognition accuracy could reach 95.20%, showing that the proposed algorithm was effective and could be used for the health status perception and disease prevention of dairy cows.
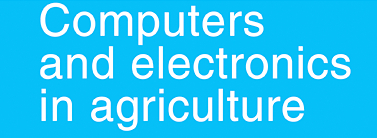