Document type: scientific article published in Computers and Electronics in Agriculture
Authors: Yue Gao, Kai Yan, Baisheng Dai, Hongmin Sun, Yanling Yin, Runze Liu, Weizheng Shen
Preview: Aggressive behavior of group-housed pigs seriously affects farm economy and animal welfare. Automatic and accurate recognition of aggressive behavior of group-housed pigs is thus important for farm production management. This study proposes a hybrid model that combines convolutional neural network (CNN) and gated recurrent unit (GRU) to differentiate aggressive and other behaviors from surveillance videos. The CNN network served as a spatial feature extractor to learn appearance representations of behavior in each individual frame, while the GRU network served as a temporal feature extractor to learn motion representations of behavior in a behavior episode. More importantly, to focus on the saliency features in both the spatial domain and the temporal domain of behavior, a specific spatio-temporal attention mechanism was designed and integrated in the CNN-GRU hybrid model to improve the effect of aggressive behavior recognition. To evaluate the proposed model, a behavior video dataset consisting of 5530 behavior episodes about 10 piglets. The accuracy of the proposed hybrid model conducted on the test set were 94.8 %. The results showed that the proposed hybrid model integrated with spatio-temporal attention performed better than the model with an independent spatial or temporal attention and the model without attention mechanism, and achieved a competitive performance of aggressive behavior recognition over the state-of-the-art approaches. We shared our behavior video dataset at https://github.com/IPCLab-NEAU/Aggressive-Behavior-Recognition for precision livestock farming research community.
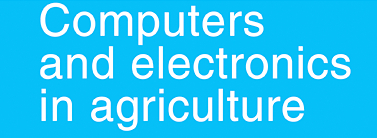