Document type : scientific article published in Computers and Electronics in Agriculture
Authors: Zishuo Gu, Haoyu Zhang, Zhiqiang He, Kai Niu
Preview: Recently, computer vision has been widely used in livestock behavior detection. In animal multi-behavior detection, there is a common problem of low recognition accuracy. To address this, a deep learning-based method for sheep-behavior detection is proposed in this paper. Six types of sheep behavior can be recognized by the method. Among these behaviors, standing, feeding, and lying are normal physiological activities. Attacking, biting, and climbing are disruptive behaviors that could result in ranch losses and require immediate concern from the breeder. The method consists of two stages: the detection stage and the classification stage. In the detection stage, a detection network is used to determine whether each sheep's behavior belongs to normal physiological activities or disruptive behaviors respectively. Based on a classic network, the multi-scale feature aggregation, attention mechanism and depthwise convolution module are imported to it, which makes the network trades off between detection accuracy and model size. These improvements make the network more suitable for sheep-behavior detection. In the classification stage, the VGG network is utilized to classify the behavior of each sheep specifically. Experimental results demonstrate that the two-stage method achieves desirable results in sheep-behavior recognition. In the detection stage, the mAP of the two types of behaviors exceeds 98%. In the classification stage, the classification accuracy of all behaviors exceeds 94%. At the same time, the memory of the detection model is less than 130 MB.
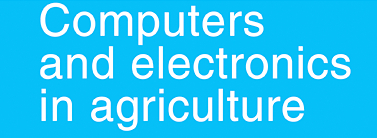